We never Had Truly Understood the Bias-Variance Trade-off!!!
In this interview with Prof. Mikhail Belkin, we will discuss his amazing paper: “Reconciling modern machine learning practice and the bias-variance trade-off” which introduces the other half of the bias-variance trade-off that we have been missing all these years. Behold the Double-Descent behavior!
In this exciting interview with Prof. Mikhail Belkin, we go over one of his publications entitled: “Reconciling modern machine-learning practice and the classical bias–variance trade-off”.
00:51 Prof. Belkin introduces himself
01:27 The research interests of Prof. Belkin
02:35 What is the story behind the paper and how did they even think about challenging the traditional single-descent U-shape plot of bias-variance trade-off?
05:40 The double-descent behavior of the interpolation models.
06:56 What if over-parametrized models did not have any regularization mechanism? Would they still generalize well (according to the double-descent behavior found in this paper)?
10:34 What is this idea of Interpolation Threshold and can it be computed mathematically?
14:32 What is Inductive Bias and how can we control it? (related to the ‘smoothness’ which is chosen as the inductive bias in the paper)
17:31 How did you determine that ‘smoothness’ is the right inductive-bias for the problems you have worked on in the paper? What if it was not? Would we still see the double-descent behavior?
25:57 The bottom of the second descent (for an over-parametrized model) does NOT necessarily correspond to less Test Error (better generalizability), compared to the bottom of the traditional U-shape model (for an under-parametrized model).
29:60 If we were not able to create highly rich models, maybe we would not have never questioned the U-shape model. What other well-founded theoretical foundation is out there (similar to the old regime and the U-shape behavior) that you think are ONLY half the picture and need us to investigate them more?
36:46 A lot of biological models out there are over-parametrized!
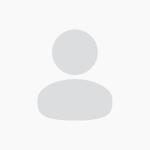
Author: Mehran
Dr. Mehran H. Bazargani is a researcher and educator specialising in machine learning and computational neuroscience. He earned his Ph.D. from University College Dublin, where his research centered on semi-supervised anomaly detection through the application of One-Class Radial Basis Function (RBF) Networks. His academic foundation was laid with a Bachelor of Science degree in Information Technology, followed by a Master of Science in Computer Engineering from Eastern Mediterranean University, where he focused on molecular communication facilitated by relay nodes in nano wireless sensor networks. Dr. Bazargani’s research interests are situated at the intersection of artificial intelligence and neuroscience, with an emphasis on developing brain-inspired artificial neural networks grounded in the Free Energy Principle. His work aims to model human cognition, including perception, decision-making, and planning, by integrating advanced concepts such as predictive coding and active inference. As a NeuroInsight Marie Skłodowska-Curie Fellow, Dr. Bazargani is currently investigating the mechanisms underlying hallucinations, conceptualising them as instances of false inference about the environment. His research seeks to address this phenomenon in neuropsychiatric disorders by employing brain-inspired AI models, notably predictive coding (PC) networks, to simulate hallucinatory experiences in human perception.
Responses